Digital Twin for HPC Engineers, Scientists and Managers
Understanding future physical componentry and its real-world behavior is required for truly advanced product development. The Rescale platform enables engineers to capture this understanding early in the development process — and to incredible levels of detail.
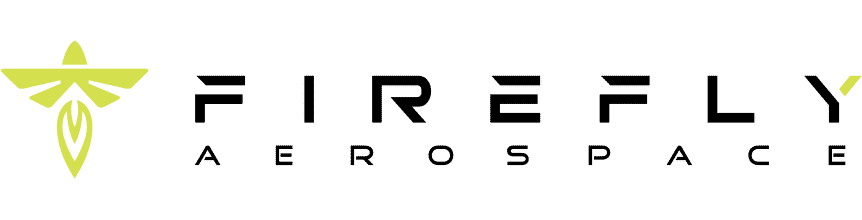
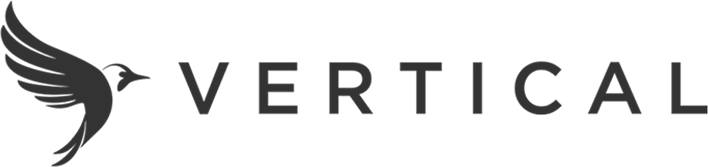

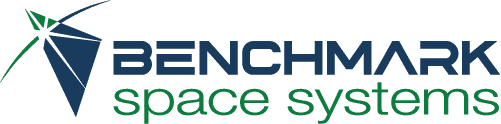
Benefits of Digital Twin in HPC
Accelerate Simulation Cycle Times
Rescale’s platform has built-in features for managing files efficiently and securely, including data transfer, version control, and file sharing.
Handle Both Simple and Complex Workflows
Go beyond simple batch jobs with built-in optimization and design of experiments frameworks.
Contents
What Is A Digital Twin?
How Does A Digital Twin Work?
What Are the Different Types of Digital Twins?
The History of Digital Twin
What Are Some of The Use Cases for Digital Twin?
What Is the Future of Digital Twin?
Want to learn more about hpc built for the cloud?

What Is A Digital Twin?
A digital twin is a virtual representation or digital model of a physical object or system that uses real-time data and other sources to mimic its real-world counterpart. Digital twins provide both the elements and dynamics of how an object operates and behaves.
Sensors can be placed on physical assets like turbines, pipelines, or manufacturing equipment to collect data on the status, condition, and performance of the system. This data is then fed into the digital twin model, which uses artificial intelligence, machine learning and analytics to process the data. The digital twin provides insights on how the physical system is currently operating, detects issues early, and runs simulations to predict future performance.
Digital twins allow companies to virtually test changes to systems or conditions without impacting actual operations. For example, they could simulate how a wind turbine would perform under various wind speeds and weather conditions. The learnings can then optimize real-world assets and operations. Digital twins are becoming a crucial component of the Internet of Things, allowing remote monitoring, controlling, and optimizing of assets from anywhere. As computing power and AI advance, so will the capabilities of digital twin technology.
How Does A Digital Twin Work?
A digital twin begins with creating a virtual model of a physical object or system. This includes defining both the physical components and the rules or physics that govern how it operates and interacts with the environment. For example, a digital twin of a wind turbine would incorporate its blades, generator, sensors, control systems, aerodynamic properties, etc.
The virtual model is then connected to the actual physical asset through an array of sensors that collect real-time data. The sensors could track temperature, vibration, airflow, throughput, or any metrics relevant to the asset’s operation. Additionally, historical data about the system can be fed into the digital twin for a more complete picture.
Powerful simulation software runs the digital twin model, leveraging artificial intelligence, machine learning, and analytics. It ingests the incoming real-world data from sensors to mirror how the physical system is performing in a virtual environment. Complex algorithms simulate the interactions between components and identify patterns and insights not obvious to humans.
Operators can then perform “what if” scenarios with the digital twin, adjusting various parameters to predict how changes may impact operations. The digital twin provides a sandbox to iterate and optimize, while minimizing disruptions to the actual system. Actions found to enhance performance can then be applied to the physical asset.
Over time, the machine learning capabilities of the digital twin model continuously improve by comparing simulations to actual results. This allows the digital representations to become increasingly precise and valuable. Digital twins combine physical world data with advanced analytics to optimize productivity, prevent downtime, and develop new opportunities.
What Are the Different Types of Digital Twins?
There are a few main types or categories of digital twins:
- Product Twin – A virtual copy of a physical product like a jet engine, wind turbine, or MRI machine. Used to optimize individual asset performance.
- Production Twin – Simulates an entire production process, such as an assembly line or oil refinery. Optimizes operational efficiency.
- Performance Twin – Models the performance of products or assets in the field. Provides insights on real-world operating conditions.
- System Twin – Represents an entire system of assets, processes, and people. Used to optimize the system holistically.
- Prototype Twin – A digital twin model created during the design phase, before a physical asset is built. Allows virtually testing prototypes.
- Composite Twin – An aggregation of various digital twins into one larger representation. Provides a broader enterprise-level view.
The type of digital twin aligns closely with its intended business purpose – either enhancing individual assets, operational processes, real-world performance, complete systems or prototypes. Some digital twins may combine elements of multiple types for different angles of insight. The key is choosing the right scope for the digital twin to effectively optimize the physical assets and business activities it mirrors.
The History of Digital Twin
The concept of digital twin has existed for decades, but has rapidly gained traction in recent years. One of the first mentions of the term was by NASA in the 1960s, when they created digital twins of spacecrafts to mirror real-time status. However, the capabilities were primitive compared to today.
Advancements in Internet of Things sensors, cloud computing, and simulation software in the 2010s enabled more complex digital twin models to emerge. GE pioneered the industrial use of digital twins for assets like jet engines and wind turbines to improve operations and maintenance. Dassault Systèmes applied digital twins across product lifecycles from design to end-use.
Over the past 5 years, the scope of digital twins has expanded enormously. Cloud platforms from Microsoft, AWS and others now provide specialized digital twin capabilities. Enterprise applications apply digital twins across facilities, supply chains, and more. Combining AI and machine learning algorithms, digital twins are achieving higher sophistication and business value. According to Gartner, over 50% of large industrial companies will implement digital twins by 2026. The digital twin technology is positioned to revolutionize many aspects of industry and manufacturing.
While digital twins emerged decades ago, recent exponential progress has unleashed their potential for organizations to gain insights, optimize operations, and improve innovations – driving considerable productivity gains across nearly every sector. We are still just scratching the surface of this emerging technology area.
What Are Some of The Use Cases for Digital Twin?
Here are some common and emerging use cases for digital twin technology across various industries:
- Predictive Maintenance – Digital twins of equipment like turbines or assembly lines can identify issues and failure risk before downtime occurs.
- Virtual Commissioning – New facilities, production lines, and other processes can be simulated and tested virtually before physical installation.
- Remote Monitoring – Digital twins enable remotely tracking assets, fleet vehicles, and more to analyze performance.
- Product Development – New product designs can be refined and optimized through simulating in a digital twin first.
- Training – High risk scenarios like medical procedures or disaster response can be simulated in a digital twin for training.
- Smart Cities – Digital twins of city infrastructure, traffic patterns, and air flows can optimize transportation and services.
- Logistics – Modeling supply chains digitally can identify optimizations, bottlenecks, and prepare for disruptions.
- Healthcare – Digital patients based on scans and data can customize treatments or medical devices.
The applications span from individual equipment optimization to organization-wide initiatives. Digital twin use cases will rapidly grow as the technology and associated benefits become more understood.
What Is the Future of Digital Twin?
The future looks very promising for digital twin technology across industries. Here are some predictions around the future of digital twins:
- Wider Adoption – Digital twins will move from limited niche uses to mainstream adoption across sectors like manufacturing, cities, healthcare, retail, and more.
- Greater Intelligence – With advancements in artificial intelligence and machine learning, digital twins will become increasingly sophisticated and insightful.
- Expanded Integration – Digital twins will incorporate more data sources like IoT sensors, business systems, and external data for comprehensive models.
- Democratization – Platforms and tools will enable more organizations to implement digital twins without deep technical expertise.
- Customization – Highly tailored digital twins will be constructed using modular libraries of pre-built components.
- Lifecycle Uses – Digital twins will expand beyond just operations to uses across the lifecycle like design, training, and end-user monitoring.
- New Business Models – Digital twin technology may spur innovative business opportunities around simulations, predictive insights, and virtual services.
- Automated Actions – Digital twins will progress from passive monitoring to automating actions, repairs, and controls of physical assets.
In summary, digital twins are poised to evolve from leading-edge to mainstream, driving dramatic gains in efficiency, performance, safety, and innovation across many industries. Their capabilities will exponentially grow with advances in enabling technologies.
Explore More With Our Team of Experts
To explore more on digital twin, please reach out to our team of experts.